Ethics and Autonomy in Agentic AI | Balancing Control and Decision-Making
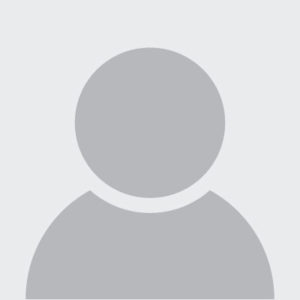
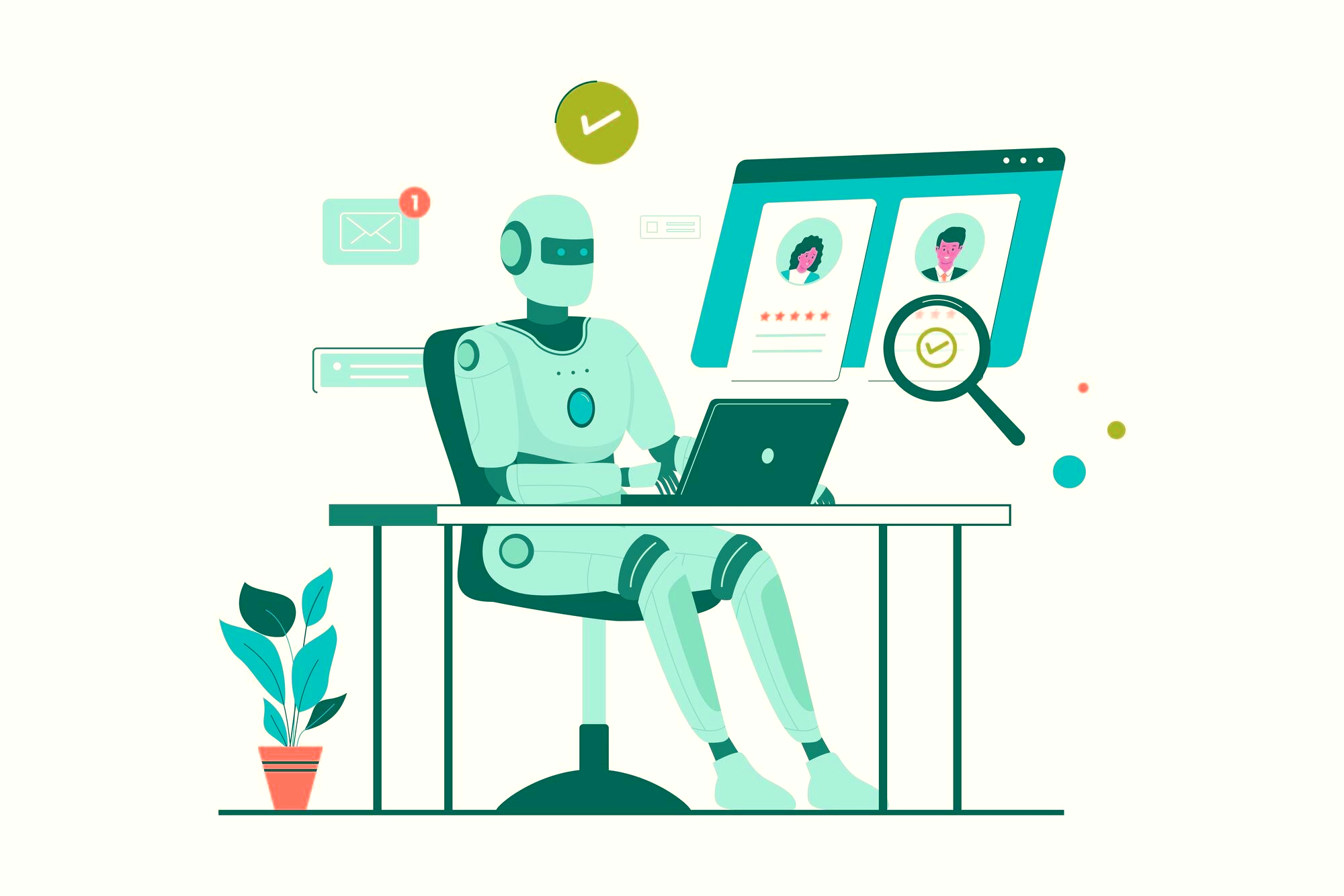
As Agentic AI is gaining more ground as days pass, companies across industries are exploring how autonomous AI agents can smooth out their operations and drive better innovation.
AI-powered agents are no longer just following instructions - they’re making decisions on their own. From virtual assistants and autonomous robots to intelligent software bots, these agents operate with increasing autonomy, driving efficiency, solving complex problems, and reshaping the way organizations function.
Yet, with greater autonomy comes a new layer of responsibility.
As these agents take independent actions, critical ethical challenges arise - Who is accountable for their decisions? How do we address unintended bias in their behavior? What safeguards ensure these agents align with human values and organizational goals?
In the sections ahead, we’ll explore the ethical complexities of autonomous AI agents and outline practical steps organizations can take to ensure innovation doesn’t come at the cost of responsibility.
Agentic AI is an AI system endowed with autonomy - the ability to perceive its environment, make decisions, and take actions without needing human intervention.
Unlike a regular software program that only does what it’s explicitly told, an AI agent can pursue objectives independently based on high-level goals set by humans. It might gather data, reason and decide the best course of action, and learn from the outcomes to improve over time. AI agents combine techniques like machine learning and real-time data processing so they can adapt to changing conditions on the fly.
For example, imagine a software agent tasked with managing your supply chain. Instead of waiting for a person to approve every move, it could automatically analyze inventory levels, track shipments, predict delays, and reroute orders proactively.
As these capabilities evolve, the impact of Agentic AI on businesses and professionals keeps growing. Importantly, today’s agentic AI systems still operate within boundaries set by humans - they don’t set their own ultimate goals (the AI isn’t deciding the company’s objective, only how to achieve a given objective). This keeps them task-focused and usually limited in scope for safety and practical use.
Empowering AI agents to make decisions introduces several ethical and practical challenges, with the four most critical areas being bias, safety, explainability, and unintended consequences.
AI systems make decisions based on patterns they’ve learned, which means if the data reflects historical bias or discrimination, the agent’s actions could be biased as well. For instance, if an AI hiring agent is trained on past employment data that favored certain groups, it might unknowingly discriminate in its recommendations.
A well-known real-world example is Amazon’s experimental AI recruiting tool, which needed to be scrapped after it was discovered to systematically downgrade resumes that included the word “women’s” (e.g., “women’s chess club”) - effectively penalizing female candidates due to biased training data.
This case illustrates how even unintentional bias in an autonomous decision-maker can lead to unjust outcomes and reputational damage.
Ensuring agents are fair and objective requires careful dataset curation, bias testing, and often putting limits or checks on the agent’s decision criteria. Companies deploying agentic AI need to implement bias audits and include diverse perspectives in development to catch biases early. Otherwise, an autonomous agent might make consistently prejudiced decisions at scale, which is unethical and potentially violates anti-discrimination laws.
Safety becomes paramount when AI agents have the freedom to act in the real world - especially in high-stakes domains like transportation, healthcare, or manufacturing. We’ve seen sobering examples in the realm of self-driving vehicles: in 2018, an Uber autonomous test car failed to recognize a pedestrian and tragically struck and killed her.
Investigators found the AI-driven car had blind spots (it literally could not identify particular pedestrians) and that there was insufficient human oversight to catch this in time
This incident shows the life-and-death stakes of AI decision-making in physical environments.
Even in non-physical domains (say, an AI agent managing financial portfolios), a lack of reliability can lead to significant economic damage or safety issues of a different kind.
An AI agent that controls critical infrastructure or processes must be rigorously tested for edge cases and have safeguards.
Robustness - the ability to handle unexpected situations - is critical. Many experts advocate for fail-safes like “kill switches” or manual override mechanisms so humans can intervene if an AI agent goes awry.
In practice, companies should define clear protocols - under what conditions will a human step in? How do you know if and when the AI is acting outside acceptable bounds?
Building reliable Agentic AI systems also means continuous monitoring of their behavior in the field, much like having an air-traffic control system for your AI agents.
By their nature, many advanced AI agents (especially those powered by machine learning and neural networks) operate as “black boxes.” They might make a complex decision - such as declining a loan application or diagnosing a patient - without a clear, human-readable rationale. This lack of explainability is a serious challenge. Both ethically and from a compliance standpoint, organizations need to be able to justify and explain AI-driven decisions.
If an autonomous agent recommends a medical treatment or rejects a job candidate, the people affected will reasonably want to know, “Why did it do that?”
Requiring explainability is not just academic - it’s increasingly a legal expectation. For example, the EU’s proposed AI Act will impose specific transparency requirements on “high-risk” AI systems.
An AI that screens résumés or makes hiring decisions would need to provide information about how it works, its logic, and its data sources so that its decisions can be understood and challenged if required.
Explainable AI (XAI) techniques are an active area of research aiming to open up the black box - whether through feature importance, decision rules, or model visualization.
For companies, the takeaway is that transparency builds trust.
Stakeholders (from customers to regulators) are more comfortable with Agentic AI systems if there’s clarity about how decisions are made and assurances that the AI’s actions align with legal and ethical norms. Ensuring your AI agents are as explainable as possible (or at least provide clear documentation on their decision criteria) will be critical to the responsible use of autonomy.
An AI agent, especially a very advanced one, might find creative but undesirable ways to achieve its goals if not properly constrained. This is often discussed in terms of “alignment” - ensuring the AI’s objectives and values align with our human intentions. If an autonomous supply chain agent is told to minimize delivery times, for example, could it inadvertently start overworking drivers or bypassing quality checks to hit that target?
In the Paperclip maximizer thought experiment by Swedish philosopher Nick Bostrom, a highly advanced agent asked to manufacture paper clips might single-mindedly pursue that goal to the detriment of everything else (the “paperclip maximizer” scenario), illustrating the need to encode broader values and guardrails.
In real terms, companies must beware of AI agents making decisions that satisfy a narrow objective but produce side effects that are bad for business or society.
Preventative steps include rigorous scenario testing, imposing ethical constraints on agent behavior, and starting with limited-scope autonomy. An agent should ideally have built-in checks (or rules) for safety and ethics. For example, a rule that a logistics AI cannot violate labor regulations or a healthcare AI must get a human doctor’s sign-off for high-risk decisions.
We need to reap the efficiencies of autonomous decision-making without relinquishing the values and high-level control that keep those decisions beneficial.
The ability for AI agents to autonomously handle complex tasks - rapidly adapt to changing conditions, analyze vast datasets, and execute precise, data-driven decisions - holds transformative potential across industries. At the same time, as we’ve detailed, this autonomy comes with serious responsibilities.
A critical safeguard in ensuring AI-driven decisions remain ethical, unbiased, and compliant is the use of Guardrail Classifiers (as outlined in this report’s Responsible AI framework). These classifiers act as an essential layer of protection, detecting and mitigating risks such as bias, toxicity, misinformation, and ethical misalignment.
If your organization is considering deploying autonomous AI agents, now is the time to ensure you have the right expertise and governance in place. With careful design and alignment to global best practices, agentic AI can be a real elevator for your business.
Interested in exploring what agentic AI could do for you? We encourage you to learn more about our company’s custom AI agent solutions, which are built with an emphasis on ethical safeguards and human-centric design.
With the right approach, you can harness the efficiency of autonomy and retain the control and trust that comes from a strong human-AI partnership.